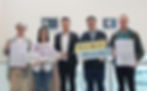
As the world grapples with the disease burden of COVID-19, The Chinese University of Hong Kong’s (CUHK) Faculty of Medicine (CU Medicine) conducted a study using a self-developed text-matching algorithm to analyse extensive amounts of free-text symptom narratives. The narratives included case-series data of COVID-19 patients reported up to 25 August 2022. The analysis delivered insights into the disease’s changing symptom profiles across COVID-19 variants and patients’ vaccination status. Notably, it identified a set of symptoms, including fever, blocked nose, pneumonia and shortness of breath, that are jointly predictive of death among unvaccinated, symptomatic, elderly patients. Study details have been published in the Journal of Medical Virology.
As Artificial intelligence (AI) large language models are increasingly important these days, the researchers conducted a parallel study to explore the possibility of popular AI large language model ChatGPT converting symptom narratives into structured data, discovering its potential in infectious disease epidemiology research. Results showed that ChatGPT could identify common symptoms from free-text narratives with a sensitivity of at least 85%. Details of this study have been published in Clinical Microbiology and Infection.
The two studies improve our understanding of COVID-19 symptoms
The first study involved an extensive analysis of free-text symptom narratives from over 76,000 COVID-19 patients, using a self-developed text-matching algorithm. Results showed that 70.9% of patients were symptomatic, with 102 symptoms identified. Researchers discovered that the wild-type and the delta variant had induced similar symptoms among unvaccinated, symptomatic patients, but the omicron BA.2 subvariant had showcased a different symptom pattern from the wild-type, with seven symptoms (fatigue, fever, chest pain, runny nose, sputum production, nausea or vomiting, and sore throat) more prevalent in the BA.2 cohort. The study also demonstrated that among symptomatic patients who had received at least two vaccine doses, BA.2 infection was more likely than delta infection to cause fever. In addition, it identified a set of symptoms, including fever, blocked nose, pneumonia and shortness of breath, that are jointly predictive of death among unvaccinated, symptomatic, elderly patients. This finding can inform strategic healthcare planning in residential care homes for the elderly.
With the analysis of free-text narratives, researchers were able to delineate a wide spectrum of symptoms. However, extracting analysable data from the free-text symptom narratives was challenging and time-consuming. Therefore, the research team explored the future landscape of AI large language model use in medical research. In the parallel study, the researchers demonstrated a methodology that ChatGPT could use to extract symptom data from free-text narratives after prompt engineering. The model was able to perform the task with high specificity of 94.7% to 100% for all symptoms, and high sensitivity of 85.3% to 100% for common symptoms.
Underscoring the role of AI large language models in efficiently structuring and decoding intricate medical narratives
Miss Vivian Wei Wan-in, Research Associate from The Jockey Club School of Public Health and Primary Care at CU Medicine, said, “By employing a self-developed text-matching algorithm, we depicted the evolution of COVID-19 symptoms across variants and vaccination status. Notably, it identified a set of symptoms predictive of death among unvaccinated, symptomatic, elderly patients, aiding residential care homes for the elderly with their targeted interventions and resource allocation. The study substantiates the role of AI large language models as a medical research tool, streamlining the conversion of complex symptom narratives into structured data. These findings pave the way for AI-driven tools to enhance early detection, monitoring and response during future pandemics.”
Professor Kwok Kin-on, Associate Professor from The Jockey Club School of Public Health and Primary Care at CU Medicine, added, “AI large language models, exemplified by ChatGPT, signifies a transformative leap in infectious disease epidemiology. These models, adept at real-time data synthesis, offer rapid insights into disease progression and early detection of emerging threats. Their ability to convert unstructured narratives into structured data streamlines decision-making and optimises resource allocation. They enhance public health communication by generating clear, comprehensible information for diverse audiences. Crucially, these models continuously learn and adapt, staying ahead of the evolving nature of infectious diseases. Their agility in processing diverse datasets at scale sets a precedent for more effective, data-driven pandemic response strategies, contributing significantly to the dynamic landscape of infectious disease research and preparedness.”
Other research team members from The Jockey Club School of Public Health and Primary Care at CU Medicine include Professor Samuel Wong Yeung-shan, Director; Professor Yeoh Eng-kiong, Professor of Public Health and Director of the Centre for Health Systems and Policy Research; Dr Cyrus Leung Lap-kwan, Postdoctoral Fellow; and Mr Edward McNeil, Senior Research Assistant. Dr Arthur Tang from Royal Melbourne Institute of Technology (RMIT) Vietnam and Professor Julian Tang from the University of Leicester and Leicester Royal Infirmary also formed part of the research team.
Details: Faculty of Medicine, CUHK: bit.ly/42XZOGN